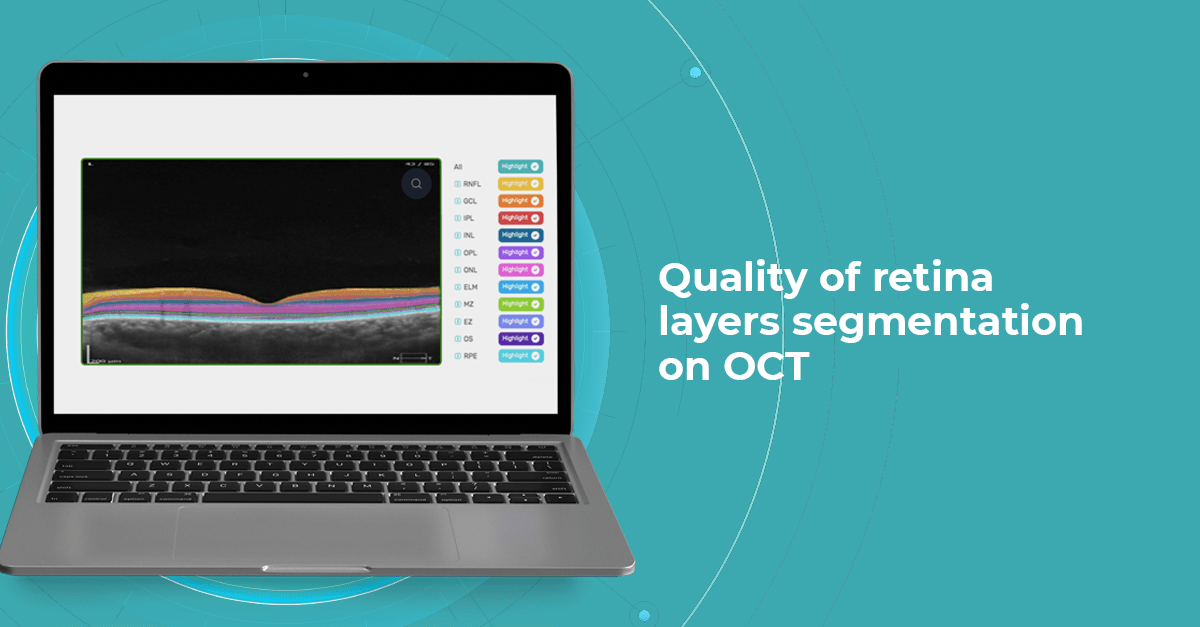
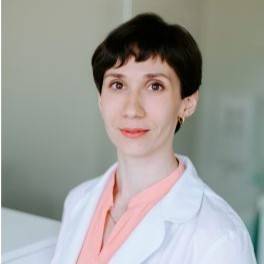
Maria Martynova
Retina Expert
Reading time
5 min.
The knowledge about macular retinal layer thicknesses and volume is an important diagnostic tool for any eye care professional today. The information about the macular retinal layers often correlates with the evaluation of severity in many pathologies.
Manual segmentation is extremely time-consuming and prone to numerous errors, which is why OCT equipment manufacturers use automatic macular retinal layer thickness segmentation.
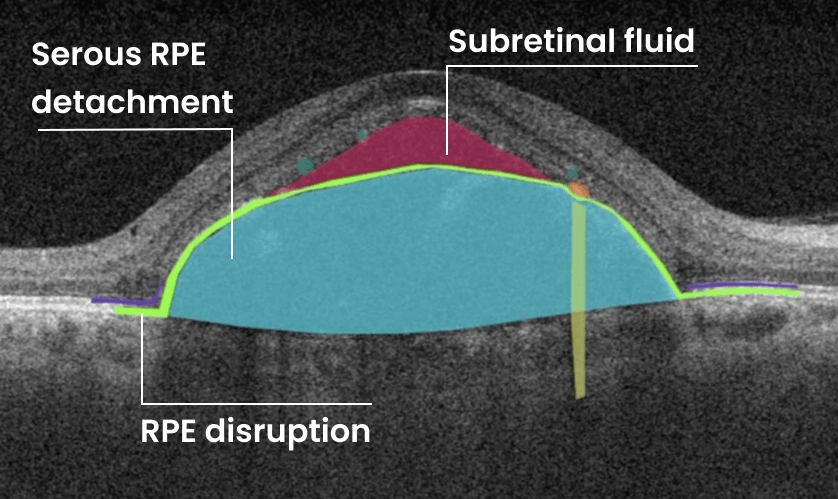
Test FDA-cleared AI for OCT analysis
Yet, retina layer segmentation in different OCT equipment manufacturers as well as in different OCT models varies significantly. It is sometimes difficult even for an experienced ECP to find the correlations and track the pathology dynamics. The normative bases refer only to the thickness of the entire retina, they are not related to segmentation. However, if the segmentation is performed incorrectly by the machine, it will lead to an incorrect calculation of the thickness of the retina or its layers, and then the assessment will be incorrect.
At Altris AI we aim to visualize retina layers for a more accurate understanding of pathological process localization. Such retina layers segmentation allows for defining the localization of the pathological process and tracing in dynamics the spread of the pathological process or the aftermath in the retina structure after its completion.
For instance, the EZ layer is important in terms of vision loss forecasting.
OCT Manufacturers & Retina Layers Analysis
From 2010 most eye care specialists have used the same OCT International Nomenclature for Optical Coherence Tomography. OCT equipment manufacturers rely on this nomenclature for retina layer thickness calculation and most ophthalmologists use it as well.
Taking into account retina structure, some layers can be united into complexes. For instance, the ganglion complex includes RNFL, ganglion cell layer & OPL.
Let’s take a look at various OCT equipment manufacturers and the way they perform retina layer segmentation analysis.
For instance, here is how Topcon Advanced Boundary Segmentation (TABSTM) automated segmentation differentiates between nine intraretinal boundaries:
- ILM
- NFL/GCL,
- GCL/IPL,
- IPL/INL,
- INL/OPL,
- ELM
- EZ
- OS/RPE
- BM
Zeiss CIRRUS uses two approaches to retina layer segmentation.
The existing segmentation algorithm (ESA) in CIRRUS estimates the positions of the inner plexiform layer (IPL) and outer plexiform layer (OPL) based on the internal limiting membrane (ILM) and retinal pigment epithelium (RPE). To improve the accuracy of the segmentation of these layers, a multi-layer segmentation algorithm (MLS) was introduced, it truly segments layers instead of estimating their position.
Heidelberg Engineering offers to learn about the following inner and outer retina layers on their website. There are 10 retina layers according to Heidelberg, and they are the following:
- ILM
- RNFL
- GCL
- IPL
- INL
- OPL
- ONL
- ELM
- PR
- RPE
- BM
- CC
- CS
Why accurate retina layer segmentation is important?
Retina layers segmentation helps eye care professionals to understand which pathology to consider in the first turn. For instance, changes in RPE and PR signify the development of Macular Degeneration.
Often such changes can also inform eye care specialists about the development of pathologies that lead to blindness, such as glaucoma, AMD, and Diabetic Retinopathy.
- Early Glaucoma Detection
Historically, evaluation of early glaucomatous change has focused mostly on optic disk changes. Modalities such as optical coherence tomography (OCT), confocal scanning laser ophthalmoscopy (HRT) or scanning laser polarimetry (GDx) with specially developed software algorithms have been used to quantitatively assess such changes. However, glaucomatous damage is primarily focused on retinal ganglion cells, which are particularly abundant in the peri-macular region (the only retinal area with a ganglion cell layer more than 1 layer thick), constituting, together with the nerve fiber layer, up to 35% of retinal macular thickness.
Therefore, glaucomatous changes causing ganglion cell death could potentially result in a reduction of retinal macular thickness. Indeed, by employing specially developed algorithms to analyze OCT scans, previous studies have reported that glaucoma, even during the early stage, results in the thinning of inner retinal layers at the macular region.
According to this study, the RNFL, GCL, and IPL levels out of all the retinal layers, the inner-most layers of the retina: the retinal nerve fiber layer (RNFL), ganglion cell layer (GCL), and inner plexiform layer (IPL) show the best discriminative power for glaucoma detection. Among these, the RNFL around the circumpapillary region has shown great potential for discrimination. The automatic detection and segmentation of these layers can be approached with different classical digital image processing techniques.
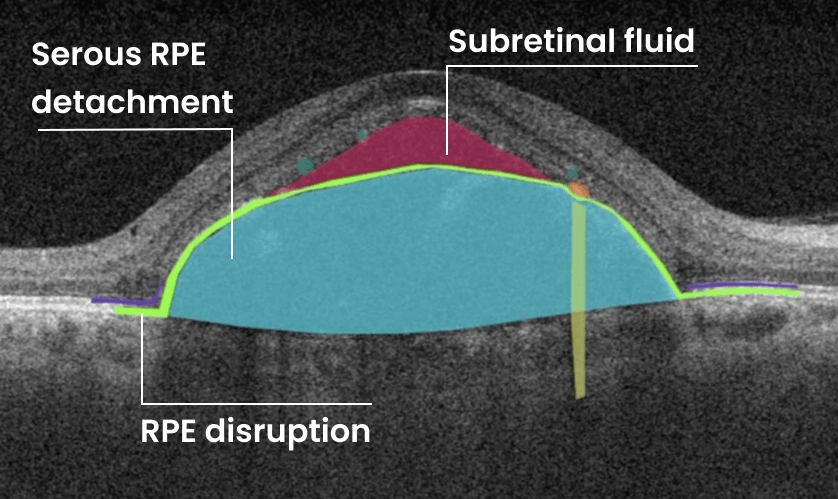
Test FDA-cleared AI for OCT analysis
- Detection of AMD
This first population-based study on spectral-domain optical coherence tomography-derived retinal layer thicknesses in a total of ∼1,000 individuals provides insights into the reliability of auto-segmentation and layer-specific reference values for an older population.
The findings showed a difference in thicknesses between early AMD and no AMD for some retinal layers, suggesting these as potential imaging biomarkers. When comparing layer thicknesses between early AMD and no AMD (822 eyes, 449 participants), the retinal pigment epithelium/Bruch’s membrane complex demonstrated a statistically significant thickening, and photoreceptor layers showed a significant thinning.
- Detection of DR
The depth and spatially resolved retinal thickness and reflectance measurements are potential biomarkers for the assessment and monitoring of Diabetic Retinopathy, one of the key reasons for blindness around the globe.
For instance, this study confirmed that decreased RNFL thickness and increased INL/OPL thickness in diabetics without DR or with initial DR suggest early alterations in the inner retina. On the contrary, the outer retina seems not to be affected at the early stages of DM. Automatic intraretinal layering by SD-OCT may be a useful tool to diagnose and monitor early intraretinal changes in DR.
Conclusion:
Retina layer segmentation is crucial for the accurate detection of pathologies in the eye, especially in the field of ophthalmology and medical imaging. Here are several reasons why it is important:
Precise Diagnosis: Retina layer segmentation provides a detailed map of the different retinal layers, which helps in the precise diagnosis of various eye conditions. It allows clinicians to identify the exact location of abnormalities, such as cysts, hemorrhages, or lesions, within the retina.
Quantitative Analysis: It enables quantitative analysis of retinal structures. By measuring the thickness, volume, and other characteristics of specific layers, clinicians can assess the severity and progression of diseases like diabetic retinopathy, macular degeneration, and glaucoma.
Early Detection: Some retinal pathologies manifest in specific layers of the retina before becoming visible on a fundus photograph. Retina layer segmentation can help detect these changes at an early stage, potentially leading to earlier intervention and improved outcomes.
Treatment Planning: Knowing the precise location of pathologies within the retina’s layers can aid in the planning of treatment strategies. For example, in cases of macular holes or retinal detachment, surgeons can use this information to guide their procedures.
Monitoring Disease Progression: Retina layer segmentation is valuable for monitoring how retinal diseases progress over time. Changes in the thickness or integrity of specific layers can be tracked to assess the effectiveness of treatments or the worsening of conditions.