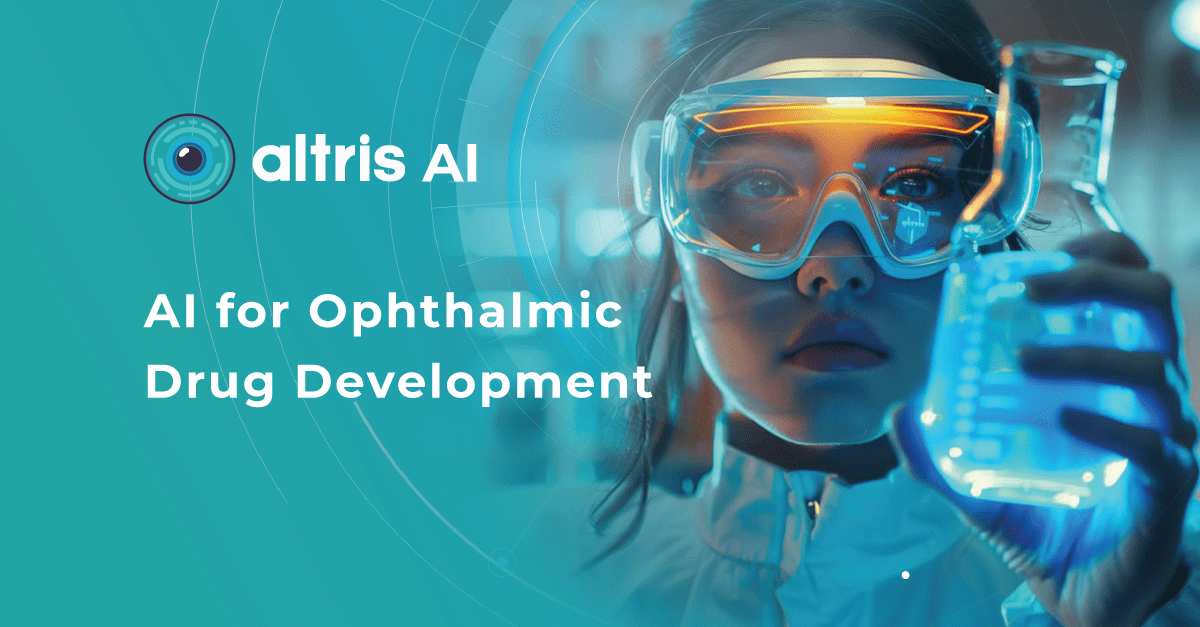
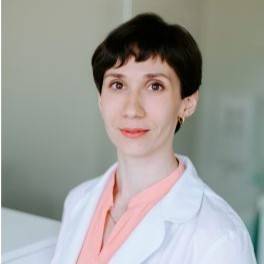
Maria Martynova
Reading time
8 min read
Despite increased research and development spending, fewer novel drugs and biologics are reaching the market today.
Large pharmaceutical companies invest an average of over $5 billion and 12+ years in research and development for each new drug approval.
The high failure rate of drug candidates (only 15% of Phase I drugs reach approval) further exacerbates the issue. This risk often leads pharmaceutical companies to favor lower-risk investments like biosimilars or generic drugs over novel therapies.
Due to the eye’s specialized anatomy and physiology, ophthalmic drug development faces unique challenges. Ocular barriers like the tear film and blood-ocular barrier can hinder drug efficacy. Many therapeutic endpoints in ophthalmology are subjective, making controlled trials difficult. The imprecise nature of some measurements further complicates trial design. Rare ophthalmic diseases pose additional challenges, as clinical trials may group diverse conditions, like multiple types of uveitic, together despite their distinct underlying mechanisms and therapeutic needs.
Here is where AI enters the game. With its ability to rapidly analyze vast amounts of data and detect subtle patterns, AI is revolutionizing how we approach clinical trials for ophthalmic drugs.
In this article, we will explore how AI for ophthalmic drug development transforms the landscape by accelerating the identification of biomarkers for conditions like diabetic retinopathy and age-related macular degeneration, ensuring the right patients are enrolled in trials, and providing quantitative metrics for evaluating treatment efficacy.
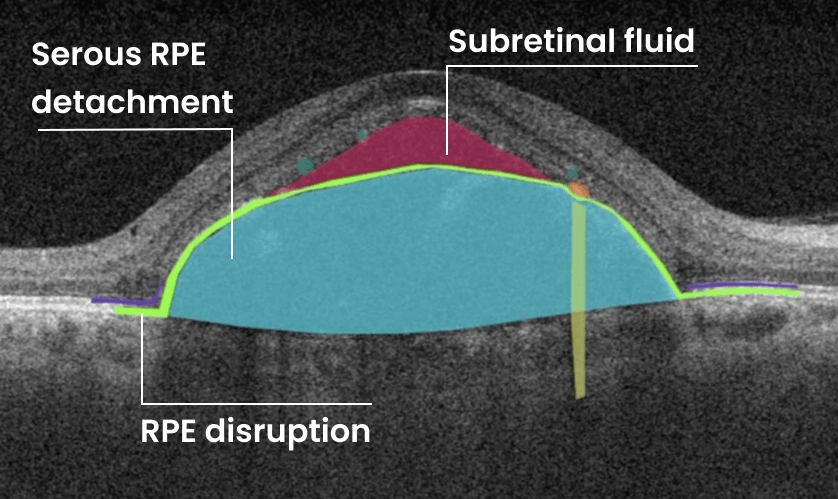
FDA-cleared AI for OCT analysis
How AI for ophthalmic drug development can accelerate the search for biomarkers in clinical trials
-
Biomarkers for quantitative analysis before and after treatment
A biomarker, as defined by the BEST Resource FDA-NIH Biomarker Working Group, is a characteristic that can be objectively measured and evaluated as an indicator of normal biological processes, disease processes, or responses to therapeutic intervention. Key characteristics of a useful biomarker include specificity, sensitivity, simplicity, reliability, reproducibility, multiplexing capability, and cost-effectiveness.
Determining a biomarker’s performance involves assessing its:
- analytical validity – how accurately it measures what it claims to measure;
- clinical validity – how well it reflects a clinical feature or outcome;
- clinical utility – how it improves patient outcomes or guides treatment decisions.
In the context of drug regulation, qualified biomarkers can serve as endpoints in clinical trials, potentially offering a more objective and less placebo-susceptible alternative to traditional patient-reported outcomes.
Imaging biomarkers are a particularly attractive option for clinical use due to their non-invasive, real-time, and cost-effective nature.
In ophthalmology, AI-powered analysis of OCT scans can provide precise, quantitative measurements of retinal thickness, fluid volume, and other biomarkers relevant to diseases like diabetic retinopathy and age-related macular degeneration. These measurements can aid in diagnosis, disease staging, treatment monitoring, and prediction of treatment response.
Systems like Altris AI for pathology detection and segmentation enabled automated disease characterization and longitudinal monitoring of therapeutic response in AMD. Multiple studies have demonstrated the value of volumetric fluid characterization, compartment-specific OCT feature evaluation, and subretinal fibrosis and hyperreflective material quantification.
A study has shown the potential of AI to predict conversion from early or intermediate non-neovascular AMD to the neovascular form, using quantitative imaging features like drusen shape and volume.
The extraction of quantitative fluid features and assessment of retinal multi-layer segmentation from OCT scans have offered valuable insights into disease prognosis and longitudinal dynamics of Diabetic Retinopathy.
A recent study demonstrated that quantitative improvement in ellipsoid zone integrity following anti-VEGF therapy for DME significantly correlated with visual function recovery. Furthermore, novel imaging biomarkers, such as the retinal fluid index (RFI), are emerging as tools for precisely monitoring treatment response. Studies have shown that early RFI volatility can predict long-term instability in visual outcomes after treatment.
Building on these advancements, researchers are now exploring the relationship between imaging biomarkers and underlying disease pathways. A recent study linked levels of various cytokines, including VEGF, MCP-1, and IL-6, with specific OCT-derived biomarkers like fluid parameters and outer retinal integrity.
By automating the analysis of OCT scans, AI not only streamlines the process but also uncovers subtle details and patterns that might be missed by human observation.
Enhanced by AI precision enables more accurate identification and quantification of biomarkers, leading to better patient stratification, treatment monitoring, and prediction of therapeutic responses.
-
Data Annotation for Clinical Trials
An ophthalmologist’s report noting the presence of edema on an OCT scan is not the same as stating that its height and length are 411 and 3213 µm, accordingly.
Imaging biomarkers can range from simple measurements of size or shape to complex computational models, providing valuable information to complement traditional diagnostic methods. They can also determine the presence and severity of a disorder, assess its progression, and evaluate treatment response.
While biomarkers can be derived from various imaging modalities, OCT stands out in ophthalmology due to its high resolution and ability to visualize subtle retinal changes.
Parametric images, which visually represent the spatial distribution of biomarker values, further enhance the analysis of OCT scans. This combination of quantitative data and visual representation empowers clinicians and researchers to make more informed decisions about diagnosis, treatment, and disease management.
Traditionally, medical image interpretation has relied heavily on visual assessment by experts, who recognize patterns and deviations from normal anatomy based on their accumulated knowledge.
While semi-quantitative scoring systems offer some level of objectivity, the field is rapidly evolving towards more quantitative and automated approaches. This shift is driven by advancements in standardization, sophisticated image analysis techniques, and the rise of machine and deep learning.
In some clinical scenarios, automated image quantification can surpass manual assessment in objectivity and accuracy, interpreting subsequent changes with greater precision and clinical relevance by establishing thresholds for disease states. Unlike physical biomaterials, medical images are easily and rapidly shared for analysis, facilitating automated, reproducible, and blinded biomarker extraction.
This transition to quantitative analysis is particularly evident in the study of AMD. For instance, non-neovascular (dry) AMD has been extensively evaluated using various imaging biomarkers, such as intraretinal hyper-reflective foci, complex drusenoid lesions, subretinal drusenoid deposits, and drusen burden.
While SD-OCT has traditionally described these features qualitatively, recent studies have demonstrated the predictive power of quantitative measures like ellipsoid zone integrity, sub-RPE compartment thickness, and automated drusen volume quantification.
These quantitative biomarkers have shown stronger associations with disease progression than qualitative features, particularly in predicting the development of geographic atrophy.
This predictive power of AI extends to diabetic retinopathy as well. In DR, quantitative measures like central subfield retinal thickness and retinal nerve fiber layer thickness have been linked to disease severity. Disruption of retinal inner layers has been associated with worse visual acuity, and its presence is highly specific for macular nonperfusion. Both DRIL and outer retinal disruption are linked to visual acuity in DR and diabetic macular edema.
Furthermore, morphological signs like hyperreflective foci, representing lipid extravasation and inflammatory cell aggregates, have emerged as potential biomarkers for monitoring inflammatory activity in diabetic eye disease. AI-powered segmentation and quantification of HRF can track changes in response to anti-VEGF and steroid injections.
-
Enrollment of the right patients
Due to their complexity and scale, clinical trials, particularly Phase III trials, consume a significant portion of the budget required to bring a new drug to the market. However, the success rate for compounds entering clinical trials is dismal, with only about one in ten progressing to FDA approval. This high failure rate stems largely from ineffective patient recruitment, as each clinical trial has unique participant requirements, including eligibility criteria, disease stage, and specific sub-phenotypes.
Manual review of electronic medical records is time-consuming and prone to error, as staff must sift through vast amounts of data to identify eligible candidates.
AI can automate this process, rapidly analyzing medical imaging and extracting relevant information to determine patient eligibility. This reduces the burden on staff and allows for faster identification and enrollment of suitable participants, streamlining patient selection and ultimately leading to more efficient and successful clinical trials.
A targeted approach can dramatically improve recruitment efficiency by pinpointing ideal candidates and even revealing disease hotspots for geographically focused efforts.
In later phases of clinical trials (Phase II and III), AI-powered image analysis can also play a pivotal role. In ophthalmology, AI can analyze OCT scans to precisely quantify disease biomarkers, ensuring that the trial participants are those most likely to benefit from the investigated drug. This improves the success rate of trials and minimizes potential harm to patients who might not be suitable candidates.
AI-powered image analysis offers a crucial advantage: reducing variability in interpretation.
AI algorithms can standardize the imaging overview process by consistently identifying and quantifying key biomarkers, ensuring that different readers arrive at similar conclusions.
-
Real World Evidence
Randomized controlled trials have long been the gold standard for evaluating the efficacy and safety of new therapies. However, controlled environments with strict inclusion and exclusion criteria may not fully reflect the diversity and complexity of real-world patient populations.
Real-world data (RWD) that is collected during routine clinical practice can provide critical insights into disease biomarkers and significantly impact the drug development process. This RWD can be transformed into real-world evidence (RWE) when appropriately analyzed.
RWE is bridging the gap between clinical trials and real-world patient care, providing a more representative view of disease progression, treatment patterns, and long-term outcomes in everyday clinical settings.
In ophthalmology, RWE already has played a crucial role in understanding the impact of anti-VEGF therapies for neovascular age-related macular degeneration. While RCTs demonstrated the initial efficacy of these treatments, RWE studies have shown variations in real-world outcomes and highlighted the need for continued and higher than previously provided treatment frequency and new treatment regimens such as treat-and-extend.
Big data, encompassing a vast array of structured and unstructured information, is now an integral part of modern medicine, including ophthalmology. By integrating RWE with traditional clinical trial data, researchers can better understand how a drug performs in the real world and conduct more pragmatic clinical trials designed to evaluate treatments in real-world settings with broader patient populations, ultimately accelerating the development of safer and more effective therapies.
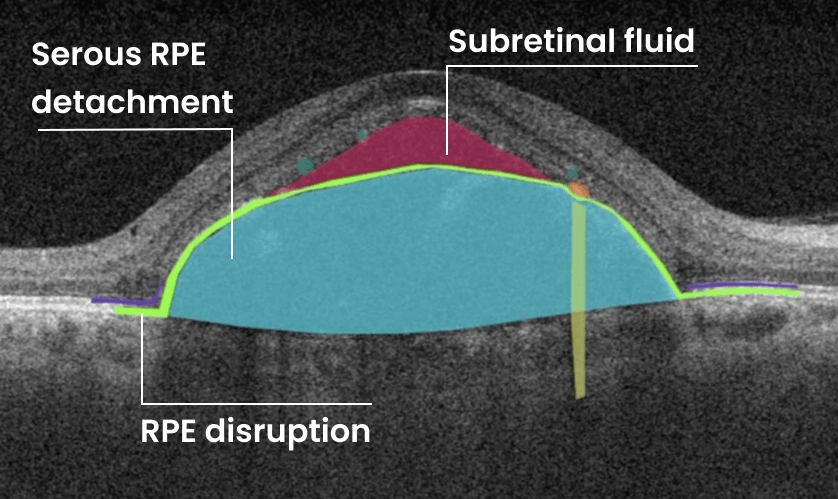
FDA-cleared AI for OCT analysis
The future of ophthalmic drug trials
The global AI-in-drug discovery market is poised for significant growth, driven by advancements in machine learning, natural language processing, and deep learning.
Artificial intelligence has the potential to significantly impact drug discovery by enabling more creative and efficient experimentation. It can also reduce the cost and time associated with failures throughout the drug development process. By identifying promising leads earlier and eliminating less viable options, AI can streamline each stage, potentially halving the total cost of a single project.
Advanced simulation and modeling techniques powered by AI are also poised to revolutionize our understanding of disease mechanisms and accelerate the discovery of new drugs.
The promising potential of AI in clinical trials extends to the proactive identification and mitigation of adverse events, enhancing patient safety and reducing trial risks. Data-driven AI tools are poised to revolutionize the entire clinical trial process, from design to execution. By streamlining patient recruitment, continuously monitoring participants, and facilitating comprehensive data analysis, AI can increase trial success rates, improve adherence, and yield more reliable endpoints.
The future of ophthalmic drug trials is here, and it’s powered by AI. By embracing this technology, researchers and clinicians can unlock new possibilities for preventing blindness and preserving vision for future generations.